By Matthew Tarnowski and Harry Thompson
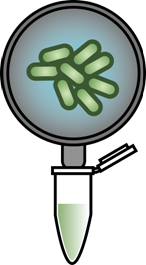
The first steps have been taken to develop a biosensor to identify specific bacteria in river water samples. Matthew Tarnowski and Harry Thompson, PhD candidates at the University of Bristol, built prototype microbe biosensors using the SHERLOCK CRISPR-based technology.
This technology relies on the ability of the CRISPR-Cas13a protein to detect specific sequences of RNA when coupled with a crRNA guide. crRNA guides can be designed to detect any unique target sequence. When the protein-RNA complex finds the target sequence, it becomes activated and begins to cleave any RNA molecules in its path, including RNA ‘reporter’ probes which ‘light up’ when cleaved.
The SHERLOCK system uses this approach to indicate the presence or absence of specific RNA sequences in samples. Please see the original publications for a schematic explaining this system in more detail [Kellner et al., (2019); Gootenberg et al., (2017)].
Producing Cas13a, a protein that can detect specific sequences of RNA
We began by transforming E. coli with the required plasmid (Addgene #90097) and then grew 8 litres of bacterial culture expressing the protein overnight (see below), before lysing the cells to release the protein. The Cas13a was then purified by affinity, ion-exchange, and finally size-exclusion chromatography methods, to yield a purified protein. This is especially important for the SHERLOCK assay, as any RNase contamination can give false positive results. The protein was then concentrated to 2 mg/mL, aliquoted and frozen at -80°C. The protein purification gel below displays the various stages of the expression and purification process. Thanks to the D40 protein gurus (Arthur, Alan and Zac, to name but a few) for all their help and advice during the purification.
Expression and purification of the Cas13a protein. (Left) The shake flasks used to grow the E. coli to express the Cas13a. (Right) SDS-PAGE protein purification gel displaying the various stages of the protein purification procedure, with protein purity increasing from left to right. (A more detailed figure is displayed at the end of this blog).
Designing crRNA target sequences
With the biosensor protein in hand, the team designed some genetic sequences that guide the protein to the target RNA, if it is present. Two computational biology tools were used: ADAPT [Metsky et al., (2022)] and BLASTN. Firstly, ADAPT was used to identify a target sequence unique to a particular organism. A house-keeping gene (rpoB) was used as a starting point, since it is commonly used as a molecular marker in microbial ecology studies [Case et al., (2017)]. Since our preliminary studies concerned the detection of a particular microorganism within mock microbial community DNA, we ran ADAPT against the rpoB sequences of other species in the community. The absence of the target sequence in other bacterial species was confirmed using BLASTN. The designed crRNA were ordered as DNA templates and then expressed using in vitro transcription, as per the SHERLOCK protocol [Kellner et al., (2019)].
Testing the biosensors
Mock microbial community DNA was used to validate the biosensor. crRNA sequences were designed and synthesised for all microorganisms found in the mock microbial community. Every design successfully gave a positive result (see below), detecting the target in the mock community sample, as intended. Furthermore, testing without the DNA present, and testing a target (bird flu) not present in the community, both gave a negative result. Some of the SHERLOCK reagents are particularly expensive and so after this first test, the assay was optimised to reduce reagent input, whilst maintaining adequate fluorescence from a positive result (results not shown). This reduced the fluorescent output of a positive result from the mock community from around 600,000 AU to around 300,000 AU.
Testing SHERLOCK biosensors against mock community DNA
The next step was to test the biosensors for cross-reaction using E. coli DNA. The results show some of the biosensors ‘light up’ in response to E. coli DNA, giving a false positive result (see below). This is despite being designed to avoid targeting other microbes in the mock microbial community. Whilst the strength of the response is weaker, testing further biosensor design iterations would be necessary to identify those that are specific to particular microorganisms.
Testing SHERLOCK biosensors against mock community and E. coli DNA
Finally, the team tested four of the biosensors against two DNA samples that had been extracted from soil. Three of the four biosensors gave a positive result for both samples (see below). The measured signal was weaker than detection of species in the mock community. This could be a result of the lower concentration of targets in the environmental DNA samples. Environmental microbiomes contain many thousands of microorganisms, which would make detection of specific species challenging. These results could be confirmed by DNA sequencing the environmental DNA samples.
Testing SHERLOCK biosensors against mock community environmental DNA (D2 & S2)
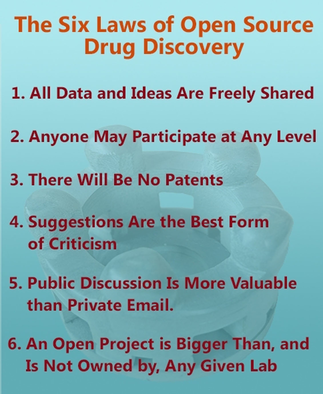
All considered, these initial results show that further optimisation is required to achieve the main goal of this ambitious project: to allow the detection of specific bacterial species in water-based samples. Whilst the biosensors detected the target species, they appeared to be susceptible to activation by RNA from other species, resulting in false positive results. This living planet is estimated to be home to one trillion microbial species, making it tricky, but not impossible, to design biosensors for the detection of particular microorganisms.
We are sharing these results to encourage a mindset of open source research, for which six guiding principles have been proposed [Todd, (2016)].
We would like to thank the SynBio CDT for the funding that facilitated this project, the D40 and Biocompute labs for the use of lab space and equipment, and Kathleen Sedgley for the help in setting up the project.
***
Supplementary
Expression and purification of the Cas13a protein. SDS-PAGE protein purification gel displaying the various stages of the protein purification procedure, with protein purity increasing from left to right. Lanes are as follows: L – protein ladder, 1 – Cleared cell lysate post sonication, 2 – Streptactin resin post binding, 3 – Streptactin resin post cleavage, 4 – Flow-through post cleavage, 5 – Concentrated sample post IEC , 6 – Concentrated sample post SEC, 7 – Diluted Cas13a aliquot (5 µL), 8 – Diluted Cas13a aliquot (1 µL). The molecular weight of SUMO-Cas13a is 155 kDa and Cas13a (post SUMO cleavage) is 139 kDa.
***
References:
Case, R. J. et al. (2007) “Use of 16S rRNA and rpoB Genes as Molecular Markers for Microbial Ecology Studies”, Applied and Environmental Microbiology, 73(1), pp. 278–288. Available at: doi.org/10.1128/AEM.01177-06.
Gootenberg, J. S. et al. (2017) “Nucleic acid detection with CRISPR-CAS13A/C2C2”, Science, 356(6336), pp. 438–442. Available at: doi.org/10.1126/science.aam9321.
Kellner, M. J. et al. (2019) “Sherlock: Nucleic acid detection with CRISPR nucleases”, Nature Protocols, 14(10), pp. 2986–3012. Available at: doi.org/10.1038/s41596-019-0210-2.
Metsky, H. C., et al. (2022). “Designing sensitive viral diagnostics with machine learning”, Nature Biotechnology, pp. 1–9. Available at: doi.org/10.1038/s41587-022-01213-5.
Todd, M. H. (2019). “Six Laws of open source drug discovery”, ChemMedChem, 14(21), pp. 1804–1809. Available at: doi.org/10.1002/cmdc.201900565.